6 January 2025
In today’s fast-paced world, managing a supply chain has become a high-stakes game. It's like spinning a dozen plates on sticks—balancing supply and demand, tracking shipments, and ensuring timely deliveries, all while dodging the curveballs thrown by unexpected events like a global pandemic or a natural disaster. That’s where machine learning (ML) steps in, almost like a backstage genius, quietly orchestrating a performance that looks seamless on the surface.
Machine learning isn’t just a buzzword anymore; it’s actively reshaping predictive supply chain management (SCM). But how exactly does it do that? Let’s break it down into digestible chunks, shall we? From demand forecasting to risk mitigation, machine learning is the secret sauce that’s revolutionizing the supply chain industry.
What Is Predictive Supply Chain Management?
Okay, before we dive into the machine learning magic, let’s get on the same page about predictive supply chain management. Simply put, predictive SCM is like having a crystal ball for your supply chain. It uses data, analytics, and forecasting models to anticipate future demands, disruptions, and trends.Think about it—if you knew that a specific product was going to experience a surge in demand two months from now, wouldn’t you start preparing today? Predictive SCM does just that! Instead of reacting to problems as they arise, it proactively helps businesses stay ahead of the curve.
But here’s the kicker: while traditional forecasting methods rely on historical data (and let’s admit, can be painfully inaccurate), machine learning takes things a step further. It analyzes vast data sets, picks up on patterns humans might miss, and makes predictions that are, frankly, borderline psychic.
The Role of Machine Learning in Predictive SCM
Machine learning isn’t just a nice-to-have in the supply chain; it’s becoming a must-have. Its ability to process enormous amounts of data and generate actionable insights is unparalleled. Let’s look at how ML is making waves in various aspects of predictive SCM.1. Smarter Demand Forecasting
Ever walked into a store only to find out they’re out of stock of the one thing you needed? Frustrating, right? Poor demand forecasting is often to blame.Machine learning can fix this. It uses algorithms to analyze historical sales data, consumer behavior patterns, seasonal trends, and even external factors like weather or economic indicators. ML doesn’t just look at raw numbers—it connects the dots.
For example, let’s say you’re a retailer, and you sell umbrellas. A traditional forecasting model might look at your past sales during rainy months. But machine learning? It goes a step further. It’ll analyze weather forecasts, economic conditions, and social media chatter about a storm. It’ll even consider regional trends, ensuring you stock just the right amount in each store.
The result? Better inventory management, reduced waste, and happier customers. Win-win!
2. Optimized Inventory Management
Excess inventory is every business owner’s nightmare. It’s like hoarding—you’re holding onto things you don’t need, which eats into your profits. On the flip side, running out of stock means missed sales opportunities. Ouch.Machine learning swoops in like a superhero here. By analyzing supply and demand patterns, ML provides real-time insights into what’s needed and when. It also factors in lead times, supplier reliability, and production capacity.
In essence, it ensures you have just enough inventory to meet demand without overstocking. Think of it as Goldilocks-level perfection—not too much, not too little, but just right.
3. Transportation and Logistics Optimization
The logistics side of supply chain management can feel like solving a Rubik’s Cube blindfolded. From route optimization to delivery scheduling, there’s a ton of moving parts.But here’s where machine learning shines. Take route optimization, for example. ML algorithms can analyze traffic patterns, fuel costs, and delivery deadlines to figure out the most efficient route. They can even factor in unexpected disruptions like road closures or accidents.
The result? Faster deliveries, lower transportation costs, and reduced carbon emissions. Can you imagine achieving all that with manual planning? Yeah, me neither.
4. Risk Management and Disruption Prediction
Let’s be real—the supply chain is vulnerable to all kinds of disruptions. From natural disasters to geopolitical events, there’s no shortage of things that can go wrong. And when they do, they create a domino effect, disrupting everything from manufacturing to delivery.Machine learning acts as an early warning system. By analyzing historical data and real-time events, it can predict potential disruptions. For example, an ML model might flag a supplier’s rising risk of default based on their financial health or geopolitical instability in their region.
This gives businesses a heads-up, allowing them to pivot, find alternative suppliers, or adjust production schedules. It’s like having a weather app that predicts storms weeks in advance, giving you enough time to grab an umbrella—or in this case, secure a backup plan.
5. Enhanced Customer Service
Let’s not forget the end customer, the person eagerly waiting for their package to arrive. Machine learning enhances customer service by improving supply chain visibility.With ML-powered predictive analytics, businesses can provide accurate delivery time estimates, proactively address delays, and even offer alternative solutions. It’s all about keeping the customer in the loop—and happy.
Ever tracked a package online and appreciated the real-time updates about its location? Yep, that’s machine learning at work.
Challenges of Implementing Machine Learning in Predictive SCM
Now, before we get carried away with the possibilities, let’s pump the brakes for a second. Like anything else, implementing machine learning comes with its fair share of challenges.1. Data Quality and Quantity
Machine learning models are only as good as the data they’re fed. Think of it like cooking—if you use bad ingredients, you’ll end up with a bad dish. Businesses need clean, accurate, and comprehensive data to train their ML models. And let’s face it, not every organization has that.2. High Implementation Costs
ML isn’t cheap. From hiring data scientists to investing in the right technology stack, the upfront costs can be daunting. But here’s the silver lining: the return on investment (ROI) can be significant in the long run.3. Resistance to Change
Let’s be honest—people don’t like change. Introducing machine learning into supply chain management often means overhauling existing processes, which can meet resistance from employees or even decision-makers.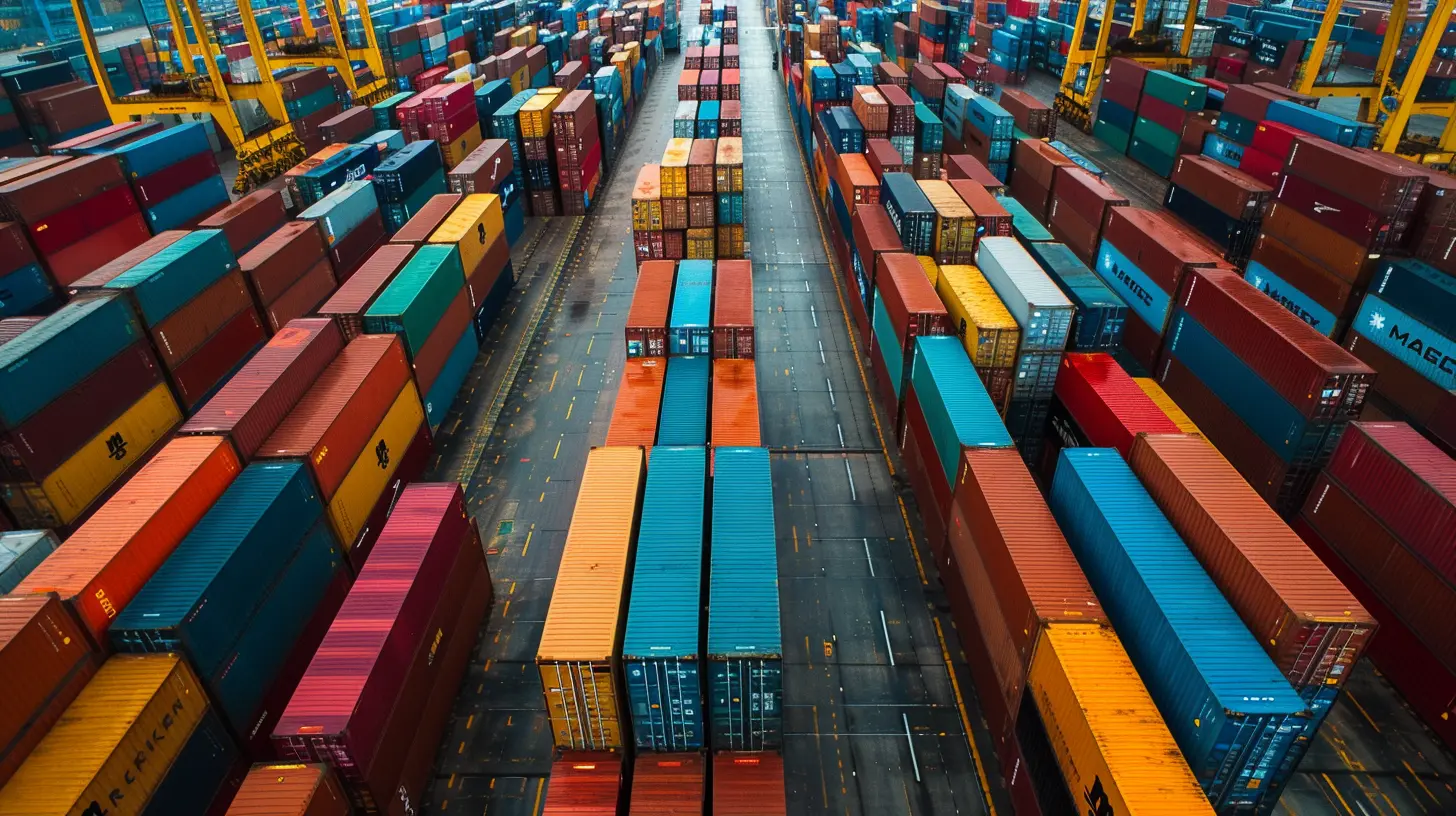
The Future of Machine Learning in Predictive SCM
So, what’s next? Well, the future is bright—and automated. As machine learning continues to evolve, its applications in predictive SCM will only grow. We’re talking about hyper-personalized customer experiences, even more accuracy in demand forecasting, and possibly autonomous supply chain operations.Imagine a world where your supply chain practically runs itself. Sure, it sounds like something out of a sci-fi movie, but with advancements in ML, it’s not too far-fetched.
Conclusion
The role of machine learning in predictive supply chain management is nothing short of transformative. From smarter demand forecasting to proactive risk management, ML is turning the unpredictable into the predictable. It’s like having a crystal ball but backed by cold, hard data.Of course, it’s not a magic wand—implementing ML comes with challenges like data quality and high costs. But when done right, the benefits far outweigh the hurdles.
So, whether you’re a supply chain manager, a business owner, or just someone fascinated by how stuff gets from point A to point B, one thing’s clear: machine learning is the future. And the future is looking pretty darn efficient.
Uzi McAllister
Ah yes, because nothing screams 'efficient supply chain' like a robot making all the decisions while we sip coffee and contemplate our life choices. Cheers to trusting machines more than our own intuition!
March 26, 2025 at 1:19 PM